Concordancer
This library loads and indexes a corpus in RAM and provides concordance search to retrieve data from the corpus with (a subset of) Corpus Query Language (CQL).
Installation
pip install -U concordancer
Usage
Concordancer is designed with this workflow in mind:
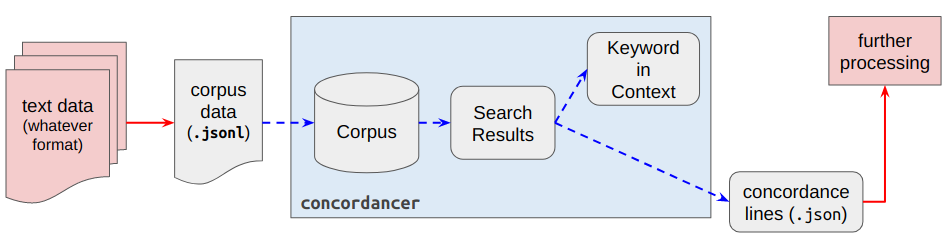
The user is expected to preprocess the text data to match the corpus data required by concordancer
. Once this is done, subsequent tasks such as indexing the copus, writing query functions to search the corpus, and displaying results in an aligned keyword-in-context format are all done by concordancer
. The user could then further process the search results (exported as JSON by concordancer
) for other uses.
Input corpus data structure
concordancer
requires the corpus to be structured (minimally) as:
[ # a corpus
{ # a text
'text': [
[<tk>, <tk>, <tk>, ...], # a sentence in a text
[<tk>, <tk>, <tk>, ...], # another sentence in a text
...
[<tk>, <tk>, <tk>, ...] # the last sentence in a text
]
},
{...}, # another text
...
]
where <tk>
is a dictionary representating a token, which may resemble something like:
{
'word': 'hits',
'lemma': 'hit',
'pos': 'V'
}
This structure allows the corpus to be saved conveniently as a newline-delimited JSON file (.jsonl
), where each line of the file corresponds to a single text in the corpus, represented as a JSON object (i.e., a dictionary in Python). You can see an example of the corpus file saved in .jsonl
here. The code below uses a corpus saved in .jsonl
format for demonstration.
Loading a corpus from file
The code below uses an example corpus, which is saved as a newline-delimited JSON file (described in the previous section).
import json
from concordancer.demo import download_demo_corpus
from concordancer.concordancer import Concordancer
from concordancer import server
# Load demo corpus
fp = download_demo_corpus(to="~/Desktop")
with open(fp, encoding="utf-8") as f:
corpus = [ json.loads(l) for l in f ]
# Index and initiate the corpus as a concordancer object
C = Concordancer(corpus)
C.set_cql_parameters(default_attr="word", max_quant=3)
Interactive Search Interface
You can start an interactive server to query and read results through your browser:
>>> server.run(C)
Initializing server...
Start serving at http://localhost:1420
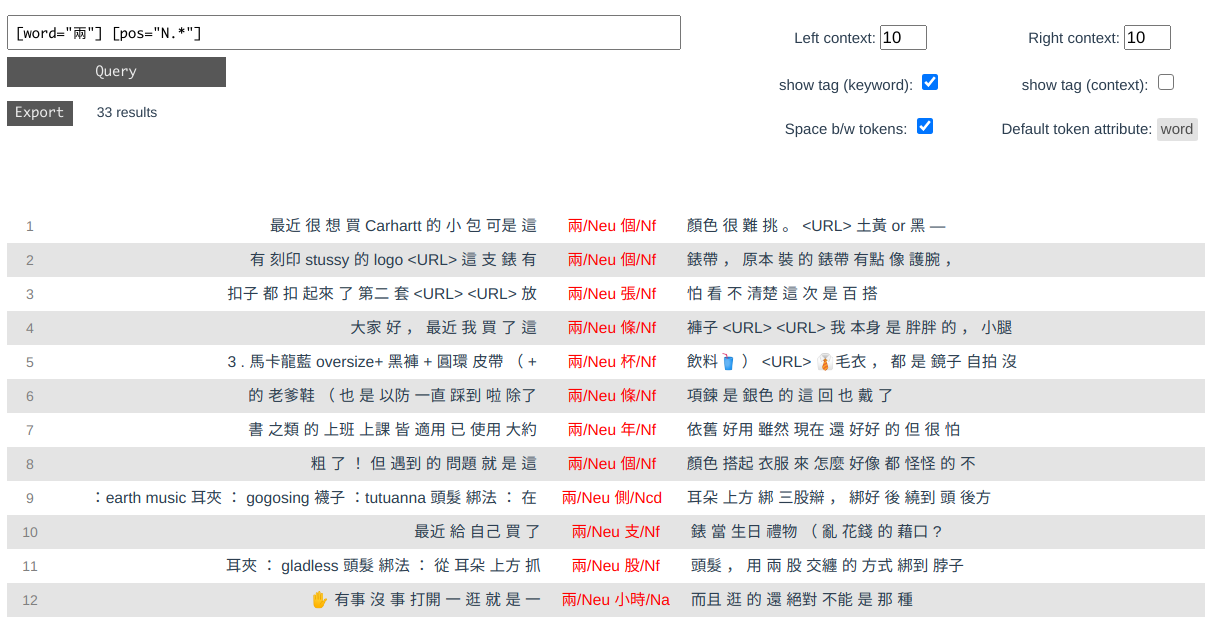
CQL Concordance search
Alternatively, you can work with the Concordancer
object, which allows you to send CQL queries to the corpus:
cql = '''
verb:[pos="V.*"] noun:[pos="N[abch]"]
'''
concord_list = C.cql_search(cql, left=2, right=2)
The results of a query is returned as a generator, which can be converted to a list of dictionaries (and then to JSON or other data structures for further uses):
>>> concord_list = list(concord_list)
>>> concord_list[:2]
[
{
'left': [{'word': '買', 'pos': 'VC'}, {'word': '了', 'pos': 'Di'}],
'keyword': [{'word': '覺得', 'pos': 'VK'}, {'word': '材質', 'pos': 'Na'}],
'right': [{'word': '很', 'pos': 'Dfa'}, {'word': '對', 'pos': 'VH'}],
'position': {'doc_idx': 78, 'sent_idx': 13, 'tk_idx': 9},
'captureGroups': {'verb': [{'word': '覺得', 'pos': 'VK'}],
'noun': [{'word': '材質', 'pos': 'Na'}]}
},
{
'left': [{'word': '“', 'pos': 'PARENTHESISCATEGORY'},
{'word': '不', 'pos': 'D'}],
'keyword': [{'word': '戴', 'pos': 'VC'}, {'word': '錶', 'pos': 'Na'}],
'right': [{'word': '世代', 'pos': 'Na'}, {'word': '”', 'pos': 'VC'}],
'position': {'doc_idx': 52, 'sent_idx': 7, 'tk_idx': 36},
'captureGroups': {'verb': [{'word': '戴', 'pos': 'VC'}],
'noun': [{'word': '錶', 'pos': 'Na'}]}
}
]
Keyword in Context
To better read the concordance lines, pass concord_list
into concordancer.kwic_print.KWIC()
to print them as a keyword-in-context format in the console:
>>> from concordancer.kwic_print import KWIC
>>> KWIC(concord_list[:5])
left keyword right LABEL: verb LABEL: noun
-------------------------- --------------- ---------------- ------------- -------------
買/VC 了/Di 覺得/VK 材質/Na 很/Dfa 對/VH 覺得/VK 材質/Na
“/PARENTHESISCATEGORY 不/D 戴/VC 錶/Na 世代/Na ”/VC 戴/VC 錶/Na
聯名鞋/Na 趁著/P 過年/VA 期間/Na 穿出去/VB 四處/D 過年/VA 期間/Na
走/VA /WHITESPACE 燒/VC 錢/Na 啊/T ~/FW 燒/VC 錢/Na
正/VH 韓/Nc 賣/VD 家/Nc 裡面/Ncd 很/Dfa 賣/VD 家/Nc
Supported CQL features
CQL search is supported through cqls, which implements a (quite useful) subset of CQL:
token:
[]
,"我"
,[word="我"]
,[word!="我" & pos="N.*"]
token-level quantifier:
+
,*
,?
,{n,m}
grouping:
("a" "b"? "c"){1,2}
label:
lab1:[word="我" & pos="N.*"] lab2:("a" "b")
Function Documentation
Example Usage